Full Paper View Go Back
Network Traffic Control Using AI
N. SelvaKumar1 , M. Rohini2 , C. Narmada3 , M. Yogeshprabhu4
1 Dept. of CSE, Coimbatore Institute of Engineering and echnology Tamil Nadu, India.
2 Dept. of CSE, Coimbatore Institute of Engineering and echnology Tamil Nadu, India.
3 Dept. of CSE, Coimbatore Institute of Engineering and echnology Tamil Nadu, India.
4 Dept. of CSE, Coimbatore Institute of Engineering and echnology Tamil Nadu, India.
Section:Research Paper, Product Type: Journal
Vol.8 ,
Issue.2 , pp.13-21, Apr-2020
Online published on May 14, 2020
Copyright © N. SelvaKumar, M. Rohini, C. Narmada, M. Yogeshprabhu . This is an open access article distributed under the Creative Commons Attribution License, which permits unrestricted use, distribution, and reproduction in any medium, provided the original work is properly cited.
View this paper at Google Scholar | DPI Digital Library
How to Cite this Paper
- IEEE Citation
- MLA Citation
- APA Citation
- BibTex Citation
- RIS Citation
IEEE Style Citation: N. SelvaKumar, M. Rohini, C. Narmada, M. Yogeshprabhu, “Network Traffic Control Using AI,” International Journal of Scientific Research in Network Security and Communication, Vol.8, Issue.2, pp.13-21, 2020.
MLA Style Citation: N. SelvaKumar, M. Rohini, C. Narmada, M. Yogeshprabhu "Network Traffic Control Using AI." International Journal of Scientific Research in Network Security and Communication 8.2 (2020): 13-21.
APA Style Citation: N. SelvaKumar, M. Rohini, C. Narmada, M. Yogeshprabhu, (2020). Network Traffic Control Using AI. International Journal of Scientific Research in Network Security and Communication, 8(2), 13-21.
BibTex Style Citation:
@article{SelvaKumar_2020,
author = {N. SelvaKumar, M. Rohini, C. Narmada, M. Yogeshprabhu},
title = {Network Traffic Control Using AI},
journal = {International Journal of Scientific Research in Network Security and Communication},
issue_date = {4 2020},
volume = {8},
Issue = {2},
month = {4},
year = {2020},
issn = {2347-2693},
pages = {13-21},
url = {https://www.isroset.org/journal/IJSRNSC/full_paper_view.php?paper_id=388},
publisher = {IJCSE, Indore, INDIA},
}
RIS Style Citation:
TY - JOUR
UR - https://www.isroset.org/journal/IJSRNSC/full_paper_view.php?paper_id=388
TI - Network Traffic Control Using AI
T2 - International Journal of Scientific Research in Network Security and Communication
AU - N. SelvaKumar, M. Rohini, C. Narmada, M. Yogeshprabhu
PY - 2020
DA - 2020/05/14
PB - IJCSE, Indore, INDIA
SP - 13-21
IS - 2
VL - 8
SN - 2347-2693
ER -
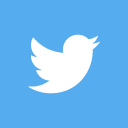
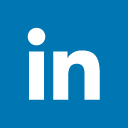
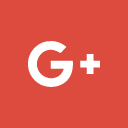
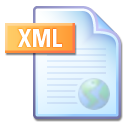
Abstract :
The core of next generation 5G wireless network is heterogeneous network. The existing traditional 4G technology approaches are centrally managed and reactive conception-based network which needs additional hardware for every update and when there is a demand for the resources in the network. To reduce traffic in 4G network techniques like Port based, payload and feature based approach are used. They do not provide efficient resource management and it does not classify all type of network traffic effectively. 5G helps in giving solution to the problem of 4G network using prediction and traffic learning to increase performance and bandwidth. Heterogeneous network provides more desirable Quality of Service (QOS) and explores the resources of the network explicitly. The assortment of heterogeneous network brings difficulty in traffic control of the network. The problem in heterogeneous network is network traffic which cannot be controlled and managed due to different protocols and data transfer rate. The key problem of heterogeneous network is to achieve intelligent and efficient internet traffic control. The upcoming 5G heterogeneous network cannot be fulfilled until Artificial Intelligence is deployed in the network. This work aims to explore artificial intelligence inspired network traffic scheme for reducing traffic in a heterogeneous network. In this work the proposed system consists of clustering of traffic data set, statistical feature extraction of the network data, prediction of network traffic and classification of network traffic. The clustering of network data is implemented using Enhanced Density-based spatial clustering of applications with noise (DBSCAN) algorithm. The statistical feature of the network data is extracted and they are given as input into the Modified Backpropagation model for prediction and regression tree method is used for classification of network traffic. Finally caching and pushing of network is included to make use of the network resource effectively and also to provide finer Quality of Service (QOS) in a network.
Key-Words / Index Term :
5GNetwork; Artificial Intelligence; Machine Learning; Data Clustering; Statistical Feature; Traffic Prediction; Traffic classification.
References :
[1] L. C. I, S. Han, Z. Xu, et al, New paradigm of 5G wireless internet [J], IEEE Journal on Selected Areas in Communications,Vol.34, No.3,474-482,2016.
[2] R. Li, Z. Zhao, X. Zhou, et al, Intelligent 5G: when cellular networks meet artificial intelligence[J], IEEE Wireless Communications, PP(99) ,2-10,2017
[3] S. Dzulkifly, L. Giupponi, F. Sai, et al, Decentralized Q learning for uplink power control[C], IEEE, International Workshop on Computer Aided Modelling and Design of Communication Links and Networks, IEEE, 54-58, 2015.
[4] M. Shafi et al.,“5G: A Tutorial Overview of Standards, Trials,Challenges, Deployment, and Practice,” IEEE JSAC, vol. 35,no. 6, , pp. 1201–21. June 2017
[5] D. Soldani, A. Manzalini, Horizon 2020 and beyond: on the5G operating system for a true digital society [J], IEEE Vehicular Technology Magazine, Vol.10, No.1, 32-42,2015.
[6] Y. Zhang et al., “Home M2M Networks: Architectures, Standards, and QoS Improvement,” IEEE Commun. Mag.,vol. 49, no. 4, Apr. 2011, pp. 44–52.
[7] Chen Z, Wen J, Geng Y. Predicting future traffic using hidden markov models. In: Proceddings of 24th IEEE International Conference on
Network Protocols (ICNP). IEEE; 2016. p. 1–6.
[8] I. Portugal, P. Alencar, and D. Cowan, “The Use of MachineLearning Algorithms in Recommender Systems: A Systematic Review,” Expert Systems with Applications, vol. 97, May 2018, pp. 205–27.
[9] S. Maharjan et al., “Dependable Demand Response Management in the Smart Grid: A Stackelberg Game Approach,” IEEE Trans. Smart Grid, vol. 4, no. 1, 2013, pp. 120–32.
[10] G. Alnwaimi, S. Vahid, and K. Moessner, “Dynamic heterogeneous learning games for opportunistic access in LTE-based macro/femtocell deployments,” IEEE Transactions on Wireless Communications, vol. 14, no. 4, pp. 2294–2308, 2015.
[11] D. D. Nguyen, H. X. Nguyen, and L. B. White, “Reinforcement Learning with Network-Assisted Feedback for Heterogeneous RAT Selection,” IEEE Transactions on Wireless Communications, vol. 16, no. 9, pp. 6062–6076, 2017.
[12] Rathidevi M and Dr. S.V . Sudha, “ Implementing Artificial Neural Network in Enhanced Micro Blog for Social Network, E Commerce and News Channels,” on International Research Journal in Advanced Engineering and Technology, Vol 3 Issue 3, ISSN: 2454-4744, E- ISSN: 2454-4752, pp. 2527-2533, 2017.
[13] U. Challita, L. Dong, and W. Saad, “Deep learning for proactive resource allocation in LTE-U networks,” in European Wireless 2017- 23rd European Wireless Conference, 2017.
[14] R. Pascanu, T. Mikolov, and Y. Bengio, “On the difficulty of training recurrent neural networks,” Tech. Rep., 2013.
[15] C. Jiang, H. Zhang, Y. Ren, Z. Han, K. C. Chen, and L. Hanzo, “Machine Learning Paradigms for Next-Generation Wireless Networks,” IEEE Wireless Communications, 2017.
[16] T. E. Bogale, X. Wang, and L. B. Le, “Machine Intelligence Techniques for Next-Generation Context-Aware Wireless Networks,” ITU Special Issue: The impact of Artificial Intelligence (AI) on communication networks and services., vol. 1, 2018
[17] G. Villarrubia, J. F. De Paz, P. Chamoso, and F. D. la Prieta, “Artificial neural networks used in optimization problems,” Neurocomputing, vol. 272, pp. 10–16, 2018.
[18] B. Bojovic´, E. Meshkova, N. Baldo, J. Riihijärvi, and M. Petrova, “Machine learning-based dynamic frequency and bandwidth allocation in self-organized LTE dense small cell deployments,” Eurasip Journal on Wireless Communications and Networking, vol. 2016, no. 1, 2016.
[19] E. Balevi and R. D. Gitlin, “Unsupervised machine learning in 5G networks for low latency communications,” 2017 IEEE 36th International Performance Computing and Communications Conference, IPCCC 2017, vol. 2018-Janua, pp. 1–2, 2018.
[20] Sadasivam Vijayakumar Sudha and Keppanagowder Thanushkodi, “ A Genetic Based Neuro Fuzzy Technique for Process Grain Sized Scheduling of Parallel Jobs,” Journal of Computer Science, pp. 48-54, ISSN 1549-3636, Science Publication 2012.
[21] U. Challita, L. Dong, and W. Saad, “Deep learning for proactive resource allocation in LTE-U networks,” in European Wireless 2017- 23rd European Wireless Conference, 2017
[22] R. Pascanu, T. Mikolov, and Y. Bengio, “On the difficulty of training recurrent neural networks,” Tech. Rep., 2013
[23] Q. V. Le, N. Jaitly, and G. E. Hinton Google, “A Simple Way to Initialize Recurrent Networks of Rectified Linear Units,” Tech. Rep., 2015.
[24] G. Alnwaimi, S. Vahid, and K. Moessner, “Dynamic heterogeneous learning games for opportunistic access in LTE-based macro/femtocell deployments,” IEEE Transactions on Wireless Communications, vol. 14, no. 4, pp. 2294–2308, 2015.
[25] D. D. Nguyen, H. X. Nguyen, and L. B. White, “Reinforcement Learning with Network-Assisted Feedback for Heterogeneous RAT Selection,” IEEE Transactions on Wireless Communications, vol. 16, no. 9, pp. 6062–6076, 2017.
[26] M. S. Parwez, D. B. Rawat, and M. Garuba, “Big data analytics for user-activity analysis and user-anomaly detection in mobile wireless network,” IEEE Transactions on Industrial Informatics, vol. 13, no. 4, pp. 2058–2065, 2017.
[27] S.V. Sudha, K. Thanushkodi, “ Use of Neural Network with Hybrid Intelligent Optimization Techniques for Weight Determination in Parallel Job Scheduling,” Scientific Research and Essays, Vol.7(26), pp.2310-2333, 12 July 2012.
[28] U. Challita, L. Dong, and W. Saad, “Deep learning for proactive resource allocation in LTE-U networks,” in European Wireless 2017- 23rd European Wireless Conference, 2017.
[29] L.-C. Wang and S. H. Cheng, “Data-Driven Resource Management for Ultra-Dense Small Cells: An Affinity Propagation Clustering Approach,” IEEE Transactions on Network Science and Engineering, vol. 4697, no. c, pp. 1–1, 2018.
[30] S. P. Sotiroudis, K. Siakavara, and J. N. Sahalos, “A Neural Network Approach to the Prediction of the Propagation Path-loss for Mobile Communications Systems in Urban Environments,” PIERS Online, vol. 3, no. 8, pp. 1175–1179, 2007.
[31] T. M. Mitchell, Machine Learning, 1st ed. McGraw-Hill Science/Engineering/Math, 1997.
[32] T. Zhou, L. Chen, and J. Shen, “Movie Recommendation System Employing the User-Based CF in Cloud Computing,” Proc. 2017 IEEE Int’l. Conf. Computational Science and Engineeringand Embedded and Ubiquitous Computing, vol. 2. 2017, pp. 46–50.
[33] P. U. Stanford Vision Lab, Stanford University, “ImageNet.” [Online]. Available: http://image-net.org/about-overview
[34] J. Erman, A. Mahanti, M. Arlitt, I. Cohen, and C. Williamson, “Semisupervised network traffic classification,” ACM International Conference on Measurement and Modeling of Computer Systems (SIGMETRICS) Performance Evaluation Review, vol. 35, no. 1, pp. 369–370, 2007
[35] A. Moore and K. Papagiannaki, “Toward the accurate identification of network applications,” in Proc. Passive and Active Measurement Workshop (PAM2005), Boston, MA, USA, March/April 2005.
[36] A. Madhukar and C. Williamson, “A longitudinal study of P2P traffic classification,” in 14th IEEE International Symposium on Modeling, Analysis, and Simulation of Computer and Telecommunication Systems, September 2006.
[37] S. Sen, O. Spatscheck, and D. Wang, “Accurate, scalable in network identification of P2P traffic using application signatures,” in WWW2004, New York, NY, USA, May 2004.
[38] J. Erman, A. Mahanti, and M. Arlitt. Byte Me: A Case for Byte Accuracy in Traffic Classification. In ACM SIGMETRICS MineNet Workshop, June 2007
[39] V. Paxson, “Empirically derived analytic models of wide-area TCP connections,” IEEE/ACM Transactions on Networking, vol. 2, no. 4, pp. 316–336, 1994.
[40] C. Dewes, A. Wichmann, and A. Feldmann, “An analysis of Internet chat systems,” in ACM/SIGCOMM Internet Measurement Conference 2003, Miami, Florida, USA, October 2003.
[41] K. Claffy, “Internet traffic characterisation,” PhD Thesis, University of California, San Diego, 1994.
[42] T. Lang, G. Armitage, P. Branch, and H.-Y. Choo, “A synthetic traffic model for Half-life,” in Proceedings of Australian
You do not have rights to view the full text article.
Please contact administration for subscription to Journal or individual article.
Mail us at ijsrnsc@gmail.com or view contact page for more details.