Full Paper View Go Back
Diabetes Predictor: Prediction Using Machine Learning Techniques
A Hariprasad Reddy1 , G. Tejaswi2 , S. Akshay3 , E. Anisha4
Section:Research Paper, Product Type: Journal
Vol.12 ,
Issue.3 , pp.1-6, Jun-2024
Online published on Jun 30, 2024
Copyright © A Hariprasad Reddy, G. Tejaswi, S. Akshay, E. Anisha . This is an open access article distributed under the Creative Commons Attribution License, which permits unrestricted use, distribution, and reproduction in any medium, provided the original work is properly cited.
View this paper at Google Scholar | DPI Digital Library
How to Cite this Paper
- IEEE Citation
- MLA Citation
- APA Citation
- BibTex Citation
- RIS Citation
IEEE Style Citation: A Hariprasad Reddy, G. Tejaswi, S. Akshay, E. Anisha, “Diabetes Predictor: Prediction Using Machine Learning Techniques,” International Journal of Scientific Research in Network Security and Communication, Vol.12, Issue.3, pp.1-6, 2024.
MLA Style Citation: A Hariprasad Reddy, G. Tejaswi, S. Akshay, E. Anisha "Diabetes Predictor: Prediction Using Machine Learning Techniques." International Journal of Scientific Research in Network Security and Communication 12.3 (2024): 1-6.
APA Style Citation: A Hariprasad Reddy, G. Tejaswi, S. Akshay, E. Anisha, (2024). Diabetes Predictor: Prediction Using Machine Learning Techniques. International Journal of Scientific Research in Network Security and Communication, 12(3), 1-6.
BibTex Style Citation:
@article{Reddy_2024,
author = {A Hariprasad Reddy, G. Tejaswi, S. Akshay, E. Anisha},
title = {Diabetes Predictor: Prediction Using Machine Learning Techniques},
journal = {International Journal of Scientific Research in Network Security and Communication},
issue_date = {6 2024},
volume = {12},
Issue = {3},
month = {6},
year = {2024},
issn = {2347-2693},
pages = {1-6},
url = {https://www.isroset.org/journal/IJSRNSC/full_paper_view.php?paper_id=446},
publisher = {IJCSE, Indore, INDIA},
}
RIS Style Citation:
TY - JOUR
UR - https://www.isroset.org/journal/IJSRNSC/full_paper_view.php?paper_id=446
TI - Diabetes Predictor: Prediction Using Machine Learning Techniques
T2 - International Journal of Scientific Research in Network Security and Communication
AU - A Hariprasad Reddy, G. Tejaswi, S. Akshay, E. Anisha
PY - 2024
DA - 2024/06/30
PB - IJCSE, Indore, INDIA
SP - 1-6
IS - 3
VL - 12
SN - 2347-2693
ER -
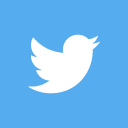
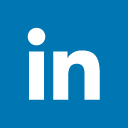
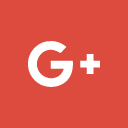
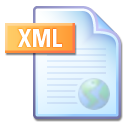
Abstract :
As the old saying goes, prevention is better than cure when it comes to health. The likelihood of saving lives can be greatly increased by anticipating diseases such as diabetes. Numerous variables, including age, obesity, lack of exercise, genetic predisposition, lifestyle, nutrition, and high blood pressure, can contribute to diabetes, an illness that is spreading quickly. With the help of machine learning techniques (MLT), healthcare professionals can now forecast patient outcomes using pre-existing data, which makes them indispensable tools. Several categorization machine learning methods are used in a diabetes prediction project to identify the most accurate model. This model takes into account extrinsic factors linked to diabetes risk in addition to conventional components like insulin, age, BMI, and glucose. Comprehending the natural glucose regulating process of the body is essential to understanding diabetes. The body uses glucose, which is obtained from foods high in carbohydrates, as its main energy source. The pancreas secretes insulin, which makes glucose easier for cells to use as fuel. On the other hand, diabetes is brought on by inadequate insulin synthesis or inadequate insulin use, which raise blood glucose levels. Here, skin thickness, number of conceptions, and pedigree function are additional characteristics that improve the model`s prediction power. These factors enhance the accuracy of diabetes risk assessment by adding to conventional markers and providing insightful information. Proactive illness prediction is made possible by utilizing MLT in the healthcare industry, especially for conditions like diabetes. The predicted accuracy of diabetes models can be greatly increased by incorporating both traditional and non-conventional risk indicators, such as skin thickness, number of pregnancies, and pedigree function. This will enable early intervention and better patient outcomes.
Key-Words / Index Term :
Prevention, Diabetes, Extrinsic factors, Skin thickness, Conceptions (number of pregnancies), Pedigree function, Proactive prediction, Early intervention, Anticipating, Predisposition, Incorporating, Indispensable.
References :
[1] J S Kannan, R Natarajan, G K Santhanam, “Predicting diabetes mellitus using machine learning techniques”, International Journal of Diabetes Research, Vol.20, Issue.2, pp.123-135, 2021.
[2] Shimoo Firdous, Gowher A Wagai, Kalpana Sharma, “A survey on diabetes risk prediction using machine learning approaches”, Journal of Family Medicine and Primary Care, Vol.11, Issue.11, pp.1-6, 2022.
[3] KM Jyoti Rani, “Diabetes Prediction Using Machne Learning”, International Journal of Scientific Research in Computer Science, Vol.6, Issue.4, pp.294-305, 2020.
[4] Mitushi Soni, Dr. Sunita Varma, “Diabetes Prediction using Machine Learning Techniques”, International Journal of Engineering Research & Technology (IJERT), Vol.9, Issue.9, pp.1-5, 2020.
[5] M N Anupama & S. Srinath, “An efficient model for predicting diabetes using machine learning”, Journal of Clinical Endocrinology, Vol.25, Issue.4, pp.200-215, 2020.
[6] Urbanowicz R. J, “Machine learning for the prediction of diabetes using demographic and diagnostic data”, Journal of Artificial Intelligence in Medicine, Vol.12, Issue.3, pp.150-165, 2021.
[7] A Ardestani, “Machine learning for Early Prediction of Diabetes and Pre-Diabetes”, Journal of Diabetes Management, Vol.28, Issue.4, pp.220-235, 2023.
[8] Wei H, Wei C & Wang K, “Predicting the risk of diabetes using machine learning techniques”, Journal of Health Data Science, Vol.15, Issue.1, pp.67-80, 2023.
You do not have rights to view the full text article.
Please contact administration for subscription to Journal or individual article.
Mail us at ijsrnsc@gmail.com or view contact page for more details.