Full Paper View Go Back
A Case Study on Brain Tumor Segmentation Using Content based Imaging
D. Sherlin1 , D. Murugan2
Section:Research Paper, Product Type: Journal
Vol.6 ,
Issue.3 , pp.1-5, Jun-2018
Online published on Jun 30, 2018
Copyright © D. Sherlin, D. Murugan . This is an open access article distributed under the Creative Commons Attribution License, which permits unrestricted use, distribution, and reproduction in any medium, provided the original work is properly cited.
View this paper at Google Scholar | DPI Digital Library
How to Cite this Paper
- IEEE Citation
- MLA Citation
- APA Citation
- BibTex Citation
- RIS Citation
IEEE Style Citation: D. Sherlin, D. Murugan, A Case Study on Brain Tumor Segmentation Using Content based Imaging, International Journal of Scientific Research in Network Security and Communication, Vol.6, Issue.3, pp.1-5, 2018.
MLA Style Citation: D. Sherlin, D. Murugan "A Case Study on Brain Tumor Segmentation Using Content based Imaging." International Journal of Scientific Research in Network Security and Communication 6.3 (2018): 1-5.
APA Style Citation: D. Sherlin, D. Murugan, (2018). A Case Study on Brain Tumor Segmentation Using Content based Imaging. International Journal of Scientific Research in Network Security and Communication, 6(3), 1-5.
BibTex Style Citation:
@article{Sherlin_2018,
author = {D. Sherlin, D. Murugan},
title = {A Case Study on Brain Tumor Segmentation Using Content based Imaging},
journal = {International Journal of Scientific Research in Network Security and Communication},
issue_date = {6 2018},
volume = {6},
Issue = {3},
month = {6},
year = {2018},
issn = {2347-2693},
pages = {1-5},
url = {https://www.isroset.org/journal/IJSRNSC/full_paper_view.php?paper_id=331},
publisher = {IJCSE, Indore, INDIA},
}
RIS Style Citation:
TY - JOUR
UR - https://www.isroset.org/journal/IJSRNSC/full_paper_view.php?paper_id=331
TI - A Case Study on Brain Tumor Segmentation Using Content based Imaging
T2 - International Journal of Scientific Research in Network Security and Communication
AU - D. Sherlin, D. Murugan
PY - 2018
DA - 2018/06/30
PB - IJCSE, Indore, INDIA
SP - 1-5
IS - 3
VL - 6
SN - 2347-2693
ER -
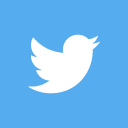
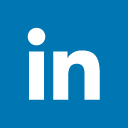
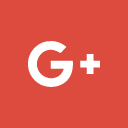
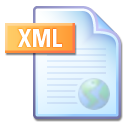
Abstract :
Brain tumors are getting to be noticeably basic in the current world for which determination of the sickness is essential as the early forecast of the malady will spare the Life. There are different kinds of tumors are there out of which gliomas are the most well-known and forceful, as the life expectancy of the individual will be decreased. For such situation culminate design about the treatment is the great move keeping in mind the end goal to enhance the patient`s life. Tumor appraisal should be possible through different ways though MRI (Magnetic Resonance Imaging) is the for the most part utilized method. Be that as it may, the disadvantage in the MRI is the measure of information produced which will set aside gigantic measure of opportunity to recognize to affirm the nearness of tumor and correct area of tumor. To stay away from this and to enhance the precision of the fragmented tumors, another method has been proposed which is Content Based Imaging Technique. In this strategy there are three distinct stages are performed specifically pre-handling, arrangement and post-preparing. The first information picture is contrasted and the arrangement of database pictures and substance are contrasted with partitioned the brain tumor as it were. Likewise the sort of tumor will likewise be distinguished alongside the precision rate.
Key-Words / Index Term :
Brain, Braintumor, Brain tumor Segmentation, Gliomas, Malignant, Content based imaging
References :
[1] S. Bauer et al., "A study of MRI-based restorative image examination for brain tumor considers," Phys. Med. Biol., vol. 58, no. 13, pp. 97β 129, 2013.
[2] D. N. Louis et al., "The 2007 who arrangement of tumors of the focal sensory system," ActaNeuropathologica, vol. 114, no. 2, pp. 97β 109, 2007.
[3] E. G. Van Meir et al., "Energizing new advances in neuro-oncology: The road to a cure for dangerous glioma," CA, Cancer J.Clinicians, vol. 60, no. 3, pp. 166β 193, 2010.
[4] G. Tabatabai et al., "Sub-atomic diagnostics of gliomas: The clinical point of view," ActaNeuropathologica, vol. 120, no. 5, pp. 585β 592, 2010.
[5] B. Menze et al., "The multimodal cerebrum tumor image division benchmark (BRATS)," IEEE Trans. Med. Imag., vol. 34, no. 10, pp. 1993β 2024, Oct. 2015.
[6] N. J. Tustison et al., "N4ITK: Improved n3 inclination adjustment," IEEE Trans. Med. Imag., vol. 29, no. 6, pp. 1310β 1320, Jun. 2010.
[7] L. G. NyΓΊl, J. K. Udupa, and X. Zhang, "New variations of a technique for MRI scale institutionalization," IEEE Trans. Med. Imag., vol. 19, no. 2, pp. 143β 150, Feb. 2000.
[8] M. Prastawa et al., "A brain tumor division system in view of anomaly identification," Med. Picture Anal., vol. 8, no. 3, pp. 275β 283, 2004.
[9] B. H. Menze et al., "A generative model for brain tumor division in multi-modular images," in Medical Image Computing and Comput.- Assisted Intervention-MICCAI 2010. New York: Springer, 2010, pp. 151β 159.
[10] A. Gooya et al., "GLISTR: Glioma image division and registration,"IEEE Trans. Med. Imag., vol. 31, no. 10, pp. 1941β 1954, Oct.2012.
[11] D. Kwon et al., "Joining generative models for multifocal glioma division and enrollment," in Medical Image Computing and Comput.- Assisted Intervention-MICCAI 2014. New York: Springer, 2014, pp. 763β 770.
[12] S. Bauer, L.- P. Nolte, and M. Reyes, "Completely programmed division of cerebrum tumor pictures utilizing bolster vector machine grouping in blend with various leveled contingent irregular field regularization," in Medical Image Computing and Comput.- Assisted Intervention-MICCAI 2011. New York: Springer, 2011, pp. 354β 361.
[13] C.- H. Lee et al., "Dividing mind tumors utilizing pseudo-restrictive irregular fields," in Medical Image Computing and Comput.- Assisted Intervention-MICCAI 2008. New York: Springer, 2008, pp. 359β 366.
[14] R. Meier et al., "A cross breed demonstrate for multimodal cerebrum tumor division," in Proc. NCI-MICCAI BRATS, 2013, pp. 31β 37.
[15] R. Meier et al., "Appearance-and setting delicate highlights for mind tumor division," in MICCAI Brain Tumor Segmentation Challenge (BraTS), 2014, pp. 20β 26.
[16] D. Zikic et al., "Choice timberlands for tissue-particular division of high-review gliomas in multi-channel MR," in Medical Image Computing and Comput.- Assisted Intervention-MICCAI 2012. NewYork: Springer, 2012, pp. 369β 376.
[17] S. Bauer et al., "Division of mind tumor pictures in light of coordinated progressive order and regularization," Proc. MICCAIBRATS, pp. 10β 13, 2012.
[18] S. Reza and K. Iftekharuddin, "Multi-fractal surface highlights for mind tumor and edema division," SPIE Med. Imag. Int. Soc. Select. Photon., pp. 903503β 903503, 2014.
[19] N. Tustison et al., "Ideal symmetric multimodal layouts and linked irregular timberlands for regulated mind tumor division (rearranged) with ANTsR," Neuroinformatics, vol. 13, no. 2, pp. 209β 225, 2015.
[20] E. Geremia, B. H. Menze, and N. Ayache, "Spatially versatile arbitrary backwoods," in Proc. IEEE tenth Int. Symp. Biomed. Imag., 2013, pp.1344β 1347.
[21] A. Pinto et al., "Mind tumor division in view of to a great degree randomized timberland with abnormal state highlights," in Proc. 37th Annu. Int. Conf. IEEE EMBC, 2015, pp. 3037β 3040.
[22] A. Islam, S. Reza, and K. M. Iftekharuddin, "Multifractal surface estimation for discovery and division of cerebrum tumors," IEEE Trans.Biomed. Eng., vol. 60, no. 11, pp. 3204β 3215, Nov. 2013.
[23] R. Meier et al., "Understanding particular semi-regulated learning for postoperative cerebrum tumor division," in Medical Image Computing and Comput.- Assisted Intervention-MICCAI 2014. New York: Springer, 2014, pp. 714β 721.
[24] Y. Bengio, A. Courville, and P. Vincent, "Portrayal taking in: An audit and new viewpoints," IEEE Trans. Example Anal.Mach. Intell., vol. 35, no. 8, pp. 1798β 1828, Aug. 2013.
[25] Y. LeCun, Y. Bengio, and G. Hinton, "Profound learning," Nature, vol.521, no. 7553, pp. 436β 444, 2015.
[26] A. Krizhevsky, I. Sutskever, and G. E. Hinton, "Imagenet grouping with profound convolutional neural systems," in Adv. Neural Inform.Process. Syst., 2012, pp. 1097β 1105.
[27] S. Dieleman, K. W. Willett, and J. Dambre, "Turn invariant convolutional neural systems for world morphology forecast," Monthly Notices R. Astronom. Soc., vol. 450, no. 2, pp. 1441β 1459, 2015.
[28] D. Ciresan et al., "Profound neural systems portion neuronal films in electron microscopy pictures," in Adv. Neural Inform. Process. Syst., 2012, pp. 2843β 2851.
[29] D. Zikic et al., "Division of brain tumor tissues with convolutional neural systems," MICCAI Multimodal Brain Tumor Segmentation Challenge (BraTS), pp. 36β 39, 2014.
[30] G. Urban et al., "Multi-modular brain tumor division utilizing profound convolutional neural systems," MICCAI Multimodal Brain Tumor Segmentation Challenge (BraTS), pp. 1β 5, 2014.
[31] A. Davy et al., "Cerebrum tumor division with profound neural systems," MICCAI Multimodal Brain Tumor Segmentation Challenge (BraTS), pp. 31β 35, 2014.
[32] M. Havaei et al., Brain tumor division with profound neural systems 2015 [Online]. Accessible: http://arxiv.org/abs/1505.03540, ArXiv:1505.03540v1
[33] M. Lyksborg et al., "An outfit of 2d convolutional neural systems for tumor division," in Image Analysis. New York: Springer, 2015, pp. 201β 211.
[34] V. Rao, M. Sharifi, and A. Jaiswal, "Cerebrum tumor division with profound learning,"MICCAIMultimodal Brain Tumor Segmentation Challenge (BraTS), pp. 56β 59, 2015.
[35] P. DvorΓ‘k and B. Menze, "Organized forecast with convolutional neural systems for multimodal cerebrum tumor division," MICCAI Multimodal Brain Tumor Segmentation Challenge (BraTS), pp. 13β 24, 2015.
[36] K. Simonyan and A. Zisserman, Very profound convolutional systems for extensive scale picture acknowledgment 2014 [Online]. Accessible: http://arxiv.org/abs/1409.1556, arXiv:1409.1556v6
[37] M. Shah et al., "Assessing force standardization on MRIs of human mind with various sclerosis," Med. Picture Anal., vol. 15, no. 2, pp.267β 282, 2011.
[38] L. NyΓΊl and J. Udupa, "On institutionalizing the MR picture force scale," Magn. Reson. Med., vol. 42, no. 6, pp. 1072β 1081, 1999.
[39] Y. LeCun et al., "Backpropagation connected to manually written postal district acknowledgment," Neural Comput., vol. 1, no. 4, pp. 541β 551, 1989.
[40] Y. LeCun et al., "Inclination based learning connected to record acknowledgment," Proc. IEEE, vol. 86, no. 11, pp. 2278β 2324, Nov. 1998.
[41] X. Glorot and Y. Bengio, "Understanding the trouble of preparing profound feedforward neural systems," in Proc. Int. Conf. Artif. Intell. Detail., 2010, pp. 249β 256.
[42] K. Jarrett et al., "What is the best multi-organize design for question acknowledgment?," in Proc. twelfth Int. Conf. IEEE Comput. Vis., 2009, pp. 2146β 2153.
[43] A. L. Maas, A. Y. Hannun, and A. Y. Ng, "Rectifier nonlinearities enhance neural system acoustic models," in Proc. ICML, 2013, vol.30.
[44] N. Srivastava et al., "Dropout: A basic method to keep neural systems from overfitting," J. Mach. Learn. Res., vol. 15, no. 1, pp1929β 1958, 2014.
[45] G. E. Hinton et al., Improving neural systems by forestalling co-adjustment of highlight identifiers ArXiv Preprint arXiv:1207.0580v1, 2012 [Online]. Accessible: http://arxiv.org/abs/1207.0580
[46] M. Kistler et al., "The virtual skeleton database: An open access vault for biomedical research and coordinated effort," J. Med. Web Res., vol. 15, no. 11, Nov. 2013.
[47] VirtualSkeleton, BRATS 2013 Sep. 30, 2015 [Online]. Accessible: https://www.virtualskeleton.ch/BRATS/Start2013
[48] F. Bastien et al., "Theano: New highlights and speed upgrades," in Deep Learn. Unsupervised Feature Learning NIPS 2012 Workshop,2012.
[49] Kalaiselvi T, Sriramakrishnan P, Somasundaram K, Survey of using GPU CUDA Programming Model in Medical Image Analysis, Informatics in Medical Unlocked, Elsevier Publications, Vol. 9, pp. 133- 144, August 2017.
[50] S. Dieleman et al., Lasagne: First Release Aug. 2015 [Online]. Accessible: http://dx.doi.org/10.5281/zenodo.27878
[51] L. R. Dice, "Measures of the measure of ecologic relationship between species," Ecology, vol. 26, no. 3, pp. 297β 302, 1945.
[52] D. Kwon et al., "Multimodal mind tumor picture division utilizing GLISTR," MICCAI Multimodal Brain Tumor Segmentation Challenge (BraTS), pp. 18, 19, 2014.
You do not have rights to view the full text article.
Please contact administration for subscription to Journal or individual article.
Mail us at ijsrnsc@gmail.com or view contact page for more details.